Scientists Turn to AI While Lawmakers Consider Guidelines
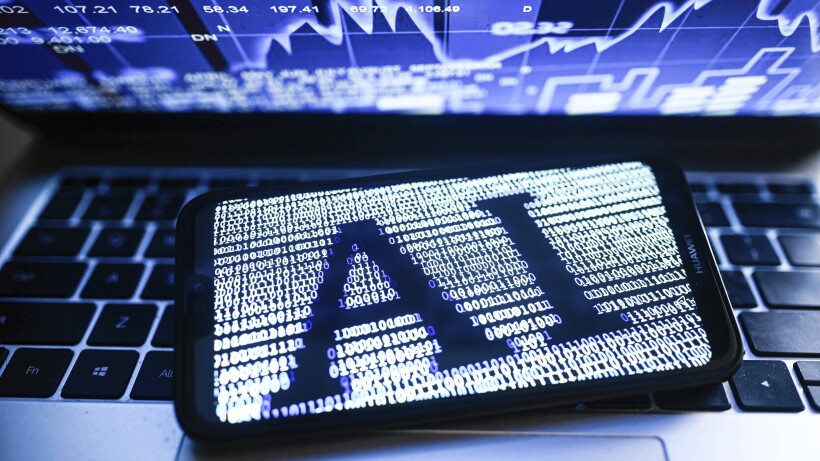
Stock illustration.
Omar Marques / SOPA Images/Sipa USA via AP
One of the primary obstacles to using AI in research is the inability to validate the accuracy of a model’s work. Lawmakers and government scientists have proposed solutions to this problem, and AI developers are chipping away at it. For example, the most recent OpenAI model, o1, was built to use a “chain of thought” that enables some insight into the processes that led to its conclusions. In the meantime, researchers are finding their own ways to use “black-box” AI and partially validate their results.
There are currently no established standards for validating the accuracy of black-box AI models, but lawmakers and scientists have expressed support for the development of such standards. In July, Sens. John Hickenlooper (D-CO) and Shelley Moore Capito (R-WV) introduced the Validation and Evaluation for Trustworthy Artificial Intelligence Act, which would require the director of the National Institute of Standards and Technology to develop voluntary guidelines for validating and evaluating AI systems.
At the same time, other lawmakers have introduced major legislation that would bolster the use of AI in science, like the Department of Energy AI Act. Researchers, federally funded and otherwise, are also moving forward in their exploration of AI as a research tool. For instance, the Climate Modeling Group at the Department of Energy, led by Peter Caldwell, is using AI models called emulators to run thousands or millions of physics-based simulations to help distinguish between climate change signals and normal weather variability.
“Maybe every 10 years or so, a new piece of technology comes along that really kind of transforms science, really accelerates things,” Caldwell said. “I think it’s clear that for this next decade, it’s AI ... I think that it’s our job as scientists in the 2020s to figure out how we can use AI, which is the technology of the moment, in order to transform our science.”
Some researchers have come up with ways to validate their machine learning models on a case-by-case basis. For a project seeking to identify topological semimetals, North Carolina State University assistant professor Milena Jovanovic and her co-authors created a model that predicts combinations of characteristics that have the strongest correlation with such semimetals but leaves the step of actually identifying topological materials to the scientists. To validate the model’s performance, the researchers used it to predict the quality of topological semimetals that were already known. Then, for unknown materials, the researchers looked at the model’s correlation map to see which characteristics and combinations the model weighed more heavily in making predictions and compared that reasoning with their own expert knowledge.
Bringing in prior knowledge helps validate the accuracy of the machine learning model, Jovanovic said. “As a chemist, you can try to intuitively explain why certain correlations would be important and why others wouldn’t … and then turn them into prediction rules that people can understand,” she said.
In her research with machine learning, Cornell University professor Eun-Ah Kim uses simple models, in part to build trust in their output, she said. It makes it easier for people to understand the reasoning behind the findings.
Kim’s first major result using machine learning used a very simple neural network to discover a new state of electronic quantum matter. “It’s such a simple model that is literally lifted from a textbook,” she said. “When the machine does all the thinking, and we cannot see how it is thinking, it might process data and speed up results, but it does not allow us to build on it.”
In discussions with DOE headquarters on how best to facilitate progress in using AI for climate science, Caldwell said he has emphasized the need for researchers to continue exploring a wide range of potential applications.
“I think that the right approach for science funding organizations right now is just to try a lot of different things,” he said. “I don’t think that it’s time to put all of our eggs in a single basket to do one kind of moonshot task.”
However, researchers’ freedom should also come with guardrails in using AI, he added.
“I don’t think that it needs regulations, but I think that it needs norms,” he said. “I think in science in general, there is so much incentive to be the first to say something provocative or new that it makes it really tempting for people to go fast and cut corners. So, I do think that there needs to be ... clear checklists and expectations that everyone needs to follow.”
Targeting the data
The proposed DOE AI Act highlights the need to aggregate, curate, and distribute AI training datasets, stating that the department has open and classified scientific datasets of “unrivaled quantity and quality” which are “a unique asset to rapidly develop frontier AI models.” The agency’s Frontiers in Artificial Intelligence for Science, Security and Technology initiative promises to transform those datasets into the world’s largest high-quality repository of AI datasets.
Louis Terminello, associate lab director at Pacific Northwest National Lab, said data availability will likely be a significant factor in the standards developed for validating AI models in different scientific domains. Existing data may not be well-curated for use in machine learning models, and model validation will likely require additional data, he said.
For Jovanovic’s study, the researchers pulled data from reported crystal structures, a topological materials database, experimental papers, and other sources. Jovanovic said the creation of additional databases where physical properties of materials are concisely reported would help in putting together a dataset for machine learning. “The biggest time sink for us is walking through literature to find out properties of materials which we’re looking at,” she said.
Furthermore, datasets are limited by human biases, Jovanovic added. “I cannot make a dataset that includes crystal structures which have never been synthesized,” she said. “This is also limited by bias in scientific literature, where people will not always publish the results of the research which has not been successful.”
In the context of scientific research, machine learning has often been employed to make relatively simple decisions about large datasets: PNNL published two studies this year that use machine learning to reduce the number of candidate materials from thousands and millions to tens and hundreds, which were much more feasible for the researchers to test.
“We were able to greatly accelerate one part of the discovery cycle,” said Terminello. “We then had to enter into a rather slow process of conventional synthesis methods… We want to accelerate that part of the discovery cycle as well, with more automated tools that are aided by AI to eventually achieve autonomy.”
In another paper, Kim and her co-authors used machine learning to analyze terabytes of data in just tens of minutes, a task that would have been prohibitively time-consuming otherwise. “My approach, generally, has been to try to leverage machines just to get over the obstacles that make it really hard for humans,” she said. “We should be automating whatever is a matter of scale.”
Jovanovic said she would like to see more creative uses of AI, not just handling problems that researchers do not have the time to tackle themselves, but those where human prediction methods do not yet exist. “The reason why one would want to use AI to predict if a material is an insulator is because it can do it faster than running a calculation. But… if AI doesn’t do it, you can do it,” she said. “I would like to see uses of AI for cases where you don’t have alternatives.”